Using machine learning as a stethoscope for 5G
The use of machine learning can identify patterns and reduce the need for human oversight—a vital means of increasing operational efficiency by reducing headcount.
June 24, 2019
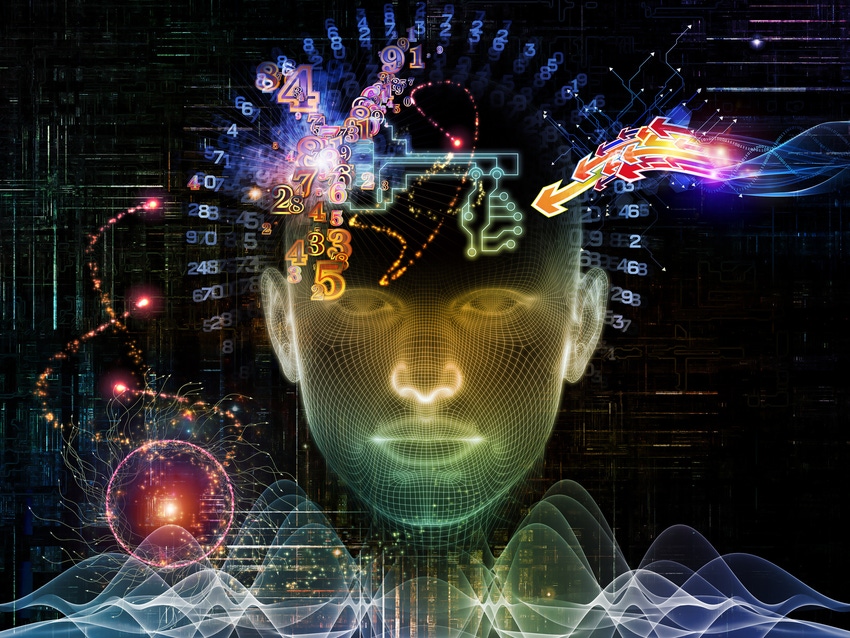
Telecoms.com periodically invites third parties to share their views on the industry’s most pressing issues. In this articleYuval Stein, AVP Technologies at TEOCO looks at the use of machine learning to optimise the roll out of 5G
The invention of the stethoscope was thanks to shyness rather than a spark of genius. In 1816, French doctor René Laennec, felt that listening to a young woman’s heart by pressing his ear to her chest wasn’t appropriate. Instead, he rolled up some paper, and found that he was able to hear much better.
The earliest designs of stethoscopes were simple wooden tubes and it was many years before the instrument we see as emblematic of healthcare was created. But the stethoscope was more than just a useful tool, it led to a new way of doing medicine. Before, it was normal to treat symptoms rather than underlying causes—now, through this new device, doctors had insight into what was going on inside the body, and were better able to understand the diseases behind the symptoms.
This shift from treating symptoms to treating causes seems natural to us now, but at this time doctors would treat a fever with no real idea of what was causing it. A similar change is now necessary with mobile networks. Increased complexity means that we need a new way of looking at these networks, to find the root causes of faults, rather than only treating the symptoms.
Machine learning as a stethoscope
The sheer amount of data that a 5G network will produce is going to be overwhelming. More data is good, of course, because the more data we have on a network, the better we can understand the issues that may be causing problems for users. But all this data needs to be analysed. In the past, this was simple—more data meant hiring more people to analyse and formulate actionable conclusions from the data.
This is no longer tenable. But it’s also no longer possible to rely on simple forms of automation in order to react to regular and more obvious issues. 5G is different from previous network generations, in that many new technologies and architectural innovations are being introduced at the same time. These technologies include NFV/SDN, edge computing, new radio access technologies and more.
This new complexity means we need new tools to examine the network. And this is where machine learning becomes just like the stethoscope—not just a tool, but a shift in how things are done. The use of machine learning can identify patterns and reduce the need for human oversight—a vital means of increasing operational efficiency by reducing headcount. But the real change is shifting from fixing issues to detecting underlying issues—even those that don’t linger in the network for long.
Treating the causes, not the symptoms
The rise of virtualised, software-driven networks has meant that service assurance is more decentralised. This means more network alarms—even with automation it’s still often impossible to determine where the real problems reside. This is particularly an issue where faults are intermittent—the symptoms may last for far longer that the fault itself. Manually examining service alarms will give an engineer no real clue as to where they can start to fix the underlying problems.
Also, there is big difference between being reactive and being proactive in maintaining a level of network assurance. A simple example would be if network bandwidth was too low to provide a certain service, and an alarm is set for when this happens. Automation would mean that the fix for this happens without any intervention. But a step further would be to use statistical techniques, such as trend analysis and forecasting to detect abnormalities in the network. These tools would mean pre-empting a situation that would result in poor service in anticipation of a glitch, rather than reacting when the issue actually arises. This isn’t about fixing problems, but preventing them before they ever happen, addressing the underlying symptoms before they have a chance to take root and cause havoc.
But machine learning can go further. Self-learning algorithms mean that operators can create a baseline profile that identifies when exceptions occur. Rather than determining a threshold for an alarm, this allows for the creation of adaptive thresholds. An example of this would be an area where many homes are being built—at some point there will be a lot more traffic in that area, but engineers don’t have the time to check how closely construction timelines are being followed. Instead, the network behavior should change to meet the demand automatically. While a hard-coded threshold would need to be reconfigured, machine learning means that thresholds are adjusted automatically.
These examples seem fairly straightforward—but millions of similar decisions need to be made every day based on an overwhelming amount of data. Operators have known that automation is necessary for some time, but machine learning is key to decision-making in a 5G network. Without it, operators will be reduced to guesswork, lacking the tools to make the most out of their new—and expensive—networks.
Yuval Stein is the AVP of Product Management and Service Assurance Products at TEOCO. With more than 15 years of experience in the service assurance domain, Yuval has held key product management positions throughout his career. He brings his knowledge to the fault, performance and service domains, and uses his hands-on experience to adapt service assurance solutions to the industry challenges: digital services and network technologies.
Read more about:
DiscussionAbout the Author(s)
You May Also Like